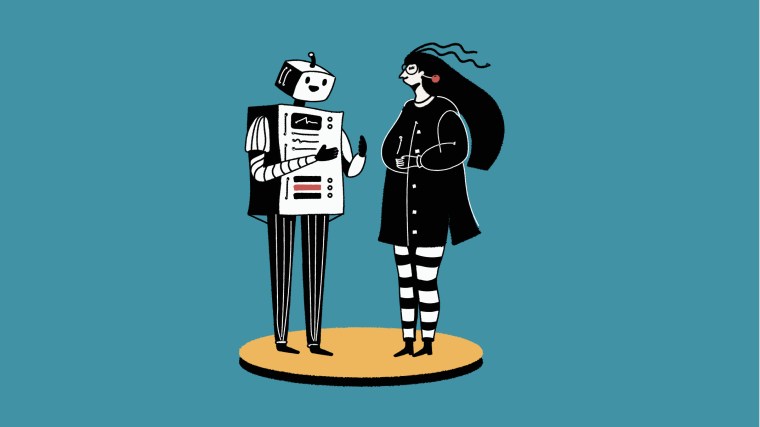
- 01 Key findings
- 02 The building blocks of personalization: In-house or vendor-supported?
- 03 Contextual customization is easier to execute, but falls short of the dream
- 04 Hyper-personalization provides precise targeting while presenting a privacy risk
- 05 Publishers skirt AI data privacy concerns by focusing on behavior
- 06 Publishers' largest use of NLP: Still chatbots
- 07 Social listening, voice-to-text translation and text enhancement use AI to accelerate workflows
- 08 Publishers largely outsource NLP application building, especially chatbots
- 09 Publishers face cost, tech and ethical challenges with AI adoption
This research is based on unique data collected from our proprietary audience of publisher, agency, brand and tech insiders. It’s available to Digiday+ members. More from the series →
This is the third part of a research series on the most popular emerging technologies. The series follows up on a report Digiday produced five years ago to discover how technologies previously reported on have evolved and to explore new technologies that have since emerged, including blockchain and robotics. In this segment, we look at how publishers are using the artificial intelligence tools of natural language processing and data-driven personalization.
Read Digiday’s 2023 report The State of AI: The paradigm shifts toward data for marketers.
More publishers have come to rely on data-driven personalization than ever before, with usage rates climbing over the past five years. In fact, publisher adoption of data-driven personalization has surged well ahead of natural language processing (NLP), according to Digiday+ Research. While both technologies fall under the larger banner of artificial intelligence, each clearly offers publishers different benefits – and exists at a different stage of maturity.
Data-driven personalization gives publishers the ability to enhance product offerings with curated content and targeted ads. Publishers have ample opportunities to use data-driven personalization to create customized user experiences. The New York Times and The Washington Post, for example, are using personalization on their homepages and apps to select and deliver content based on readers’ interests. Automated content recommendation was expected to be the most important use of AI in newsrooms in 2022, according to Statista.
NLP helps publishers enable smoother workflows and production processes with applications like social media listening, text enhancement (e.g. headline writing), voice-to-text translation and chatbots for customer service. Voice-to-text translation still has the clearest near-term publisher utility, as it can accelerate news reporting and distribution through automated transcription of audio interviews and live news events.
Ad position: web_incontent_pos1
However, NLP in the form of chatbots may gain more publisher interest as software improves, according to Vincent Cirel, chief technology officer at Gannett. “It [faced] the historical challenges of linguistics, various accents and things like that,” said Cirel. “But over the last five years, companies that specialize in [these services] have largely, I won’t say completely, conquered those problems. It’s opening business up to explore what are the great use cases for natural language processing.”
Indeed, chatbot use increased during the pandemic as businesses of all kinds relied on them to interact with consumers when in-person operations were closed. And overall uptake of AI increased too, with 52% of companies accelerating their AI adoption plans due to COVID-19, according to PwC.
With publishers already finding successful uses for data-driven personalization and NLP, and with the technologies improving, adoption of AI among publishers is bound to accelerate.
Ad position: web_incontent_pos2
For this report, Digiday+ Research surveyed 388 industry professionals including publishers, agencies, brands and retailers to uncover how they’re currently using data-driven personalization and natural language processing — and how they plan to incorporate the technologies in the future.
- Publishers have increased their prioritization of data-driven personalization in the last five years, with 62% of respondents saying it is a priority in 2022, versus 56% in 2017.
- Publishers are evenly split on whether to target an individual user for a hyper-personalized experience, or to create a customized experience based on contextual information for a more widely appealing experience.
- When it comes to gathering data and creating personalization tools, publishers most often use a mix of in-house and third-party solutions.
- Publishers focus on how, where and what users search for online in order to gather data points upon which to build audience segments. They seek to define the “who” of a user based on behaviors, rather than user-specific data, to prepare for a cookieless future.
- Data-driven personalization is becoming a must-have for publishers, with 76% of publishers that do not currently use this form of personalization saying they plan to invest in it in the future.
- Unlike data-driven personalization, publisher usage of NLP, such as chatbots, has remained roughly the same over the last five years. Forty-two percent of publishers use NLP in 2022, versus 47% in 2017.
- Chatbots are the most-used NLP technology, with 52% of publisher respondents saying they use them. But newer NLP formats, like social media listening (used by 48% of publishers) and voice-to-text (used by 42%), are closing in and could help increase overall usage of the technology.
- NLP is more evenly split than data-driven personalization when it comes to future usage. Forty-six percent of publishers who do not currently use NLP say they will invest in the technology, while 51% say it is not relevant to them.
Before publishers can get to personalizing either the content or the ad experience, they first have to lay a foundation by collecting or acquiring data and constructing their tools. To accomplish both of these things, publishers most often use a mix of in-house solutions and third-party vendors.
Digiday’s survey found that 43% of publishers jointly use in-house and third-party options to gather data, and 45% use both methods in conjunction to build applications for data-driven personalization. Exclusive use of third-party options followed for both data gathering and building applications, while depending solely on in-house solutions was the least common method.
The trend toward using a combination of in-house and third-party solutions was evident when Digiday asked publishers how they’re collecting data for personalization. Respondents said they’re primarily collecting customer first-party data (68%), which is typically gathered in-house when users register or sign up for subscriptions and provide personal information, or as they reveal their preferences through their ad and content clicks.
Web analytics tools, like Google Analytics, came in second for gathering data for personalization at 58%: These third-party applications are used to track, review and measure activity on a website. Forty-five percent of respondents also said they use third-party data sources, like Nielsen, as main information providers.
Representing the in-house camp, Forbes built its own internal data center dubbed “Forbes One” that it uses to collect and analyze first-party reader data, including content consumption behavior. “Machine learning [ML] and AI are at its core,” said Vadim Supitskiy, Forbes’ chief technology officer. “The platform allows us to do multiple things. For one, it allows us to understand our content using ML, AI and NLP, and it gives us an ability to categorize the content and understand the sentiment of the content and topics.”
Supitskiy said the data center also gives Forbes the ability to understand its audience within the context of three audience segments: behavioral, demographic and Forbes’ communities. “Using ML models and AI, we can understand what our audience wants and we can scale our audience segments,” he said. “We can create lookalike audiences at scale.”
Seventy-five percent of publisher respondents to Digiday’s survey said they use data-driven personalization for ad experiences. The New York Times’ in-house data science team built the publication’s own contextual classifications of content for ad targeting, which include the emotional tenor of a story, the topics of articles readers view and the motivations audiences feel after reading an article. They placed classification tags on The Times’ content to further identify reader demographics and interests, allowing marketers to tailor ads accordingly. Previously, The Times’ advertisers had been targeting ads through section and keyword segments.
In addition to ad targeting, 75% of publishers also said they’re using data-driven personalization for determining content or product prioritization, such as homepage layout.
Reach, a U.K.-based publisher with more than 200 publications, launched a tool called Mantis Contextual that collects data on the interests of people who visit Reach’s websites. Terry Hornsby, Reach’s group digital director, said that, ideally, publishers should be able to provide personalized content that is tailored to a reader’s short-term needs, like a sports score, and longer-term, evergreen interests.
“Our endgame would be to kind of keep evolving the personalization so that people see the content that they have come for, or that they would like,” he said. “We want to get to a point with a page where this is ‘home’ — this is where all my content is. I’ve told you I like American football, NBA and [TV show] ‘Made in Chelsea’ — and then we go: ‘Here’s all that.’ That’s where we want to get to.”
Reach is also one of several publishers using data to personalize newsletters with the hope of improving open rates, click-through rates and page visits. In 2021, The Telegraph launched its Headlines newsletter, which uses an algorithm to send personalized, vertical-specific content recommendation emails, to much success. A Telegraph spokesperson said that Headlines converts registered users to subscribers at twice the rate of its other newsletters.
However, not all publishers have been as lucky. The Toronto Star recently shuttered its personalized newsletter, first distributed in 2020. According to David Topping, newsroom director, newsletters, at Toronto Star’s owner Torstar, most subscribers “seem pretty happy getting what everyone else got” and tailored recommendations weren’t “necessarily something that’s going to move the needle.”
Publishers have increased prioritization of data-driven personalization in the last five years, thanks in part to its usefulness for making product decisions and providing audience profiles for ad targeting — both of which are revenue drivers. Sixty-two percent of publisher respondents to Digiday’s survey said data-driven personalization is a top priority in 2022 – six percentage points more than in 2017.
Another reason publishers have increased the use of data-driven personalization is because they can use personalized data to curate relevant editorial content for readers, with the goals of increasing the volume of new readers and improving engagement and retention of existing readers. The longer readers spend on a page, the more time publishers have to serve them ads, potentially increasing ad clicks and conversions.
However, when it comes to tailoring creative, editorial content and product assortment for readers, publishers are evenly split on whether to target the page context based on tags and other semantic data (50%) or the user with a hyper-personalized experience based on individual data (50%).
Contextual personalization broadly targets users by gathering information from the page a reader is viewing, rather than collecting specific information about the users themselves, to recommend related content. The most common examples of contextually curated content are “you might also like” or “people also read” sections on a page. These sections display articles tied to a reader’s assumed interests based on topics they’re currently viewing and are intended to entice users to click on additional similar stories, leading to higher engagement and more time spent on the site.
Contextual targeting is generally easier for publishers to implement than hyper-personalized targeting for a number of reasons. Hyper-personalization requires a more finely-tuned automated system since the personalization is done dynamically at an individual level, while contextual targeting uses pre-populated webpages. Contextual also doesn’t require users to provide specific personal information during sign-up or through surveys (thus skirting some privacy concerns), and publishers don’t have to invest as heavily in first-party data collection to do things like meticulously log and analyze individual users’ consumption data. And because it also reassures ad buyers of privacy compliance by default, advertisers that may have considered contextual targeting too imprecise in the past are reconsidering it.
But there are clear downsides to not being able to hyper-personalize an experience to a user, and publishers content to stick with contextual run the risk of being left behind. IPG Media Lab’s executive director Adam Simon said Netflix, for example, is known for its contextual recommendations, rather than user-specific suggested content.
“We used to talk about how great [Netflix’s] recommendations were, but they haven’t evolved as fast,” said Simon. “It’s one of those things that might start to become a differentiator in the streaming space — how you surface new content to users, what those interfaces look like, and how smart [streaming services] are about recommending what [users] should watch next when they finish a show or movie.”
Hyper-personalized experiences, on the other hand, are highly customizable and can conform dynamically to customer traits and preferences. Feed-based content, like that offered by Instagram and TikTok, is hyper-personalization’s high water mark. Instagram shows users posts based on their activity, including their connections and what posts they have liked, saved or commented on, among other things. Users can also tell Instagram if they don’t want to see a suggested post and the post will be removed from future feed suggestions, further increasing the algorithm’s intimate knowledge of the individual.
For most publishers, the dream experience social feeds represent is out of reach. But other, more accessible hyper-personalized experiences are based on information a user has willingly provided to a publisher during activities like account sign-up or via surveys. Once reluctant to dabble in personalization, The New York Times has waded further in since Digiday asked the publisher about it five years ago.
“The Times has historically been allergic to personalization,” said Andrew Phelps, who in 2017 was a member of The Times’ research and development team and is now an independent consultant. “There’s something very special about that shared experience of The New York Times: I’m looking at the same thing as the president, my congressman or somebody halfway around the world. That’s not something we ever want to do away with. But what we’re realizing now is that we can preserve that special, shared experience and, beyond the front page, create much more tailored, relevant experiences.”
Now, The New York Times recommends specific articles and pages for readers based on their individual account data, including a reader’s stated interests, geographic location and reading history, offering an effective example of hyper-personalization that is not feed-based. In March, The Times also created an “experiments and personalization” team to test personalizing the homepage of the publisher’s website and app for each reader based on their location or reading history.
Publishers like Forbes use the data they collect to create audience profiles, which they provide to advertisers so marketers can create auto-targeted ad experiences. The profiles can be used to advertise to specific pre-existing customer bases and to entice newer consumer groups.
“We can connect our advertising partners with the audiences that they are looking to reach,” said Forbes’ Supitskiy. “[An] advertiser will come to us and say, ‘We’re looking for [a specific] type of audience. And we’ll provide those audiences, but we’ll also provide insights that say, ‘Audiences that really engage with your advertising are also people who like to travel, or are interested in pets and like technology.’ So, it allows us to understand our audiences, what they interact with, how they behave, and how they react to different types of advertising and content.”
But hyper-personalization does raise some significant privacy concerns, since it requires users to reveal personal data like browsing history and demographic information that is stored and managed by the publisher. Additionally, as IPG Media Lab’s Simon noted, many platforms are not taking the next step and asking consumers for feedback about whether hyper-personalization efforts are effective.
“Very few companies are forming a good feedback loop of consumer input into that personalization,” he said. “Once you design an algorithm to personalize an experience, you should want consumers to give you feedback on how well that’s working. You can tell it you don’t want to see a specific ad, but not a specific post in the algorithmic feed. You can block or mute somebody, but you can’t mute posts from one person about specific topics…That is the next evolution — incorporating constant feedback and trying to make algorithms understand why you don’t want certain things to show up in your feed.”
Across the board, data-driven personalization also needs to surmount limitations to current automation capabilities to see wider publisher adoption. While certain portions of the experience can be dynamically tailored, such as homepage layout, other portions must still be manually updated in the backend of the website, like manually tagging articles with topics. And publishers are simply not able to manually personalize experiences for thousands of readers.
In 2022, almost half of publisher respondents (48%) said they need to use a combination of both manual and automated updates to personalize content. Only 28% of respondents said that content personalization is fully automated, while 25% said it must be completely manually updated. That doesn’t sound like a scalable solution quite yet.
As a cookieless future approaches, albeit slowly — Google delayed depreciation of the third-party cookie again until 2023 — and more stringent data privacy laws are enacted, publishers are weighing how to best provide personalized content and AI-driven experiences while respecting user privacy.
“For data privacy concerns, you’ve got two really different domains,” said Gannett’s Cirel. “One is what’s considered best practices. What are consumers comfortable with? What are the societal morays that drive that? That is a qualitative viewpoint of data privacy, versus the quantitative side, which is regulatory and legal…to fuel personalization in a way that doesn’t violate privacy and to make sure the data that you’re gathering for machine learning models does not violate data privacy laws and regulations and morals.”
Consumers’ own conflicting desires for more personalized content, but with disclosure around when and how data are collected, stored and used, must be considered as well, according to IPG Media Lab’s Simon. “The conversation around privacy and how data is used has shifted a lot,” he said. “The pivotal thing to think about is that people do want things that are more personalized, even with apps with content feeds. People do want them to be more personalized, but they want there to be some transparency.”
Indicative of the need to address privacy concerns, Digiday’s survey found that publishers and brands alike are focusing on creating new audience segments that emphasize users’ site behaviors (e.g. clicks, time on page and cart abandonment rate) over demographics. Seventy-two percent of publisher respondents said they look at site behavior as a main attribute around which to personalize editorial content, ad creative and other aspects of the user experience. That data point is followed by browser/search history (59%), indicating that publishers are primarily focused on how, where and what users are searching for online. They are placing less emphasis on demographic information like user location and device, which came in third and fourth.
Although publishers have increased their focus on site behavior, the overall list of user attributes they consider for personalization has remained largely the same since 2017. Standard “who” data points, such as age, sex and occupation, are still of value, but are used much less frequently than top data points like site behavior and browser/search history. During the last five years, the usage percentage of those customary “who” data points has remained about the same, with the exception of occupation, which had the largest decrease by about 14 percentage points.
Forbes’ Supitskiy said the publisher has worked around directly targeting users by creating audience segments based on site behavior. “Privacy is at the forefront, so we make sure to focus on that,” he said. “That was a driving factor for us, especially with third-party cookies going away. We wanted to focus not on identifying and targeting a specific user, but targeting the segment in a very privacy-forward way. We are strong believers, especially in the future, that targeting segments is going to be the way because it’s all anonymous…Definitely staying ahead of the privacy concerns and making sure that we take them into consideration.”
Hand-in-hand with privacy questions are concerns about how much personalization is too much when it comes to serving readers curated content. A reader’s media diet has the potential to become an echo chamber in which salient, but not necessarily preferred stories, are buried within an individual’s news feed due to an emphasis on personalization. Audiences may miss out on important news stories as a result.
“The flip side of that and one of the things we have to talk about when discussing AI, particularly if you’re driving personalization, is how to get bias out of AI,” said Gannett’s Cirel. “How do we recognize it?…When it comes to AI, and particularly machine learning as a subset of AI, the outputs are only as good as the data you provide to the machine. You have to have really good and well-thought-out learning models. What data do you want to feed the engine to make sure that you’re getting relevant output?”
While publishers have escalated use of data-driven personalization in the last five years, their usage of NLP applications — primarily in the form of chatbots — has remained roughly the same, with a slight decrease from 47% in 2017 to 42% in 2022. Unlike in 2017, the broader NLP category considered in our 2022 survey includes multiple technologies, like social media listening, voice-to-text translation and text enhancement. But chatbots are still the most prevalent form of NLP used by publishers, with 52% of publisher respondents saying they use chatbots in 2022.
Outside of some experiments in content delivery, publishers were initially attracted to chatbots mainly as a way to engage with and gain new audiences. Business Insider, since renamed Insider, embraced chatbots early on to send alerts about top stories to Facebook Messenger users, potentially reaching a larger and younger audience of new subscribers.
“We’re engaging our readership in a platform that they’re already seeming to migrate to and congregate,” said John Ore, then svp of product at Business Insider and now chief product officer at Insider. “The initial implementation is to provide readers with more conversational updates throughout the day rather than be a duplication of the News Feed.”
On the commercial side, chatbots give potential (and existing) subscribers immediate access to 24/7 customer service to ideally get issues resolved and questions answered. Gannett’s Cirel said chatbots not only can provide smooth consumer interactions, but have the added benefit of reducing publisher expenses.
“The best customer service operations are low friction,” he said. “If your definition of customer service is that you have to speak to another person on the other end of the line, that gets into resource constraints…But if you’ve got AI-backed natural language processing capability, so that when a consumer calls in there’s a 90% chance that they’re going to get their question answered without ever having to interact with a human being, that’s good for everybody. It keeps costs low.”
Over time, publishers have expanded their use of chatbots beyond customer service and audience building to include content distribution. The Wall Street Journal maintains a Messenger chatbot that gives readers access to breaking news and daily digests. In 2019, BBC News Labs built a “Climate Bot” chatbot to test whether it was easier for readers to understand complex topics like climate change when content is broken into several messages, rather than a traditional longer-form article.
While chatbots are still the most prevalent form of NLP used by publishers, more diverse applications are closing in and could broaden usage of the technology. Social media listening and voice-to-text are a close second and third to chatbots in terms of use cases for NLP, at 48% and 42% of publishers using them, respectively. Text enhancement follows in fourth at 36%.
Social media listening tracks user conversations on social media platforms for discussions about a brand, a company or specific topics. According to Forbes’ Supitskiy, the publisher monitors subject matter trending across the web, including on social media, and uses the findings “to notify journalists that write about similar topics” and on “a Slack channel that we push the trending information to, including content categories and sentiment of the trending topics.”
Voice-to-text is another form of NLP that tends to be used mostly internally by publishers. Reporters use it to transcribe audio interviews for faster news reporting, and it can be used to transcribe live news events so text versions of speeches and press conferences can be made available to readers more immediately. The technology enhances internal workflows by augmenting or accelerating human output. However, a downside is that translations can be incorrect due to factors like misinterpreted regional accents and colloquialisms, technical terminology, and words that have multiple meanings and spellings. Most AI-translated text can’t be published in its raw form and still needs a human eye to review and tweak it first.
Text enhancement usages for NLP came in fourth behind voice-to-text among Digiday’s survey respondents, with 36% of publishers saying they use the technology for that purpose. Steve Croll, group vp of technology at Huge, a digital agency focused on design and innovation, said the commercialization of NLP for text enhancement is particularly relevant for publishers. “We can see innovations in NLP, like [the online content writing tool] copy.ai for copywriting and how we can use AI to spark creativity,” he said.
“You don’t have to start with a blank page anymore,” Croll added. “You can describe what you want, a large language model will write it and you can be the editor instead. The model can generate 10 different versions of an article and the writer can splice it together. NLP can operate as an enhancement to the way that we do work today.”
Forbes uses text enhancement within its CMS “Bertie,” named after its founder B.C. Forbes, to analyze and recommend headline improvements, and to suggest related articles and images for stories. “It’s powered by the same insights and AI models that our journalists and contributors use to make educated, data-driven decisions,” said Forbes’ Supitskiy. “So, it’s a full circle full loop that we provide, constantly trying to provide the data, advice and insights to writers.”
Turner Sports previously used text enhancement through third-party vendor WSC Sports’ AI platform to extract video clips for Turner’s content team. In 2018, Bloomberg Media was using AI to write one-sentence summaries of news articles that had been selected for readers using personalized curation. And, as far back as 2017, The Washington Post, Associated Press and USA Today were using AI for reporting.
Gannett currently uses natural language generation (NLG), a subset of NLP that converts structured data into text, to turn data into locally relevant stories. Kara Chiles, svp of consumer products at Gannett, said the goal is to deliver targeted content to readers and grow subscriptions.
“Natural language generation techniques inform readers and reduce newsroom toil by creating uniquely localized content only possible through automation,” said Chiles. “We have the ability to zoom in on hyperlocal trends, highlighting specific local data, creating specific stories for local markets. We don’t believe NLG should replace human reporters telling compelling stories. The NLG tools help us fill gaps and provide journalists more flexibility to pursue things that can’t be automated.”
Chiles said story templates are written by reporters, shared across USA Today’s more than 230 newsrooms and reviewed by editors before being published. “This is important to us to ensure quality and that we uphold our journalistic standards,” she said. “We’re not using automated story generation to create most of our content. We analyze and edit the stories to ensure continued relevance.”
Unlike data-driven personalization applications, which publishers use a mix of in-house and third-party options to build, NLP applications are largely built by outside vendors. Fifty-eight percent of respondents said they use a third-party source, and only 15% said they use in-house applications.
Publishers who use third-party applications mainly do so for chatbots. Chatbots are the most common application of NLP and are still mostly deployed through Facebook Messenger, with 41% of respondents saying they use Messenger for chatbots.
Messenger’s chatbot application launched in 2016 and drew a lot of initial interest from businesses that used it to provide customer service, commerce options, product discovery and entertainment. Digiday found that 88% of publishers were using it for chatbot deployment in 2017. However, interest declined shortly thereafter when Facebook revealed that bots failed to respond to about 70% of requests.
To combat decreasing use of its Messenger chatbot application, Facebook added new features to the service in 2019 that were intended to increase lead generation and commerce. Capabilities included letting businesses send users to Messenger by swiping up on Stories ads or clicking on ads that go straight to Messenger. Burger King, for example, used the service to give customers the ability to plan a meal and pay for it through Messenger. Facebook’s efforts seem to have worked to some degree, as almost half of publishers said they are still using Messenger for chatbot applications in 2022.
Forbes is one of the publishers in the 15% minority that brought its NLP tools in-house, though its focus has not been chatbots. Forbes’ Supitskiy said by using its Forbes One in-house application, the company has been able to build out hundreds of custom audiences and gain a better understanding of the publication’s readers. Essentially, Forbes blends data-driven personalization, using alternate ID technology, with NLP to achieve better-informed audience results.
“We generally use the ID taxonomy, so all of our content is separated and categorized into 700-plus different ID categories,” Supitskiy said. “But with NLP, it’s important to understand what the content is about … what topics are positive and what topics are negative. We take that information and apply it to our audiences and to understand how a user segment perceives that content.
“Then we see over time what users engage with and divide that into different behavior segments. That plays a big part in the experiences and recommendations we provide. It all goes back into that engine that allows us to provide specific, personalized bespoke experiences or content recommendations for our users.”
As noted earlier, Forbes also uses the data it gathers via its in-house platform to provide advertisers with consumer segment data for ad targeting.
Five years ago, in Digiday’s previous iteration of the emerging tech series, IPG Media Lab’s Simon (then its strategy director and now executive director) said, “Not enough brands are investing in personalization and AI or machine learning.” However, that sentiment has shifted with time and companies are now prioritizing AI, both in the forms of data-driven personalization and NLP.
Of the two, data-driven personalization has clearly become a must-have for publishers, with 76% of publishers that do not currently use personalization saying they plan on investing in it in the future. Publishers have found success using data-driven personalization to both curate relevant news content for readers – potentially driving new paid subscriptions while retaining the interest of existing audience members – and to create audience segments for advertisers to use in ad targeting.
But publishers say a main barrier to wider-scale adoption of data-driven personalization is the cost of building and implementing the tools. Expenses can be especially high with the majority of publishers using in-house options in tandem with third-party partnerships to gather data and build applications for subsequent personalization. In-house tools can be costly since they often require funding separate teams with specific expertise in the technology.
On the other hand, future publisher use of NLP is more evenly split, with 46% of respondents who do not currently use NLP saying that they will invest in the technology going forward, and 51% saying it is not relevant to their business.
Publisher concerns about NLP’s usefulness was especially apparent when publishers who do not currently use NLP cited lack of relevance as their primary reason for not investing in NLP (40%). That was followed by both the cost of building and implementing the technology and lack of technical skills (each at 20%) and a lack of customer interest, a close fourth at 18%.
Because NLP tends to be used most often as a customer-facing tool in the form of chatbots, it may have more relevance to consumer brands that need to provide responses to product questions and complaints. But, even when publishers have a strong need to use chatbots for customer service or to deliver news content, chatbot technology can lag behind user expectations.
IPG Media Lab’s Simon said the tool’s usefulness can be limited by the capacity to have a smoothly flowing dialogue. “We’re finding that NLP is not advancing as fast as we want it to with things like voice assistants,” he said. “Our ability to have natural conversations with them really is happening very incrementally. It seems clear that it’s going to be probably another decade before you can just start talking to Google or Siri or or Alexa the way that you would talk to a human.”
Gannett’s Cirel said he envisions a future where it’s hard to distinguish between a bot-based conversation and one with another person. “Ideally, you want natural language processing to be developed to the point that a human being on one end of a conversation does not know, or cannot easily ascertain, that they’re not talking to a human being on the other end,” he said. “The power of natural language processing is really coming from those machine learning models, and the data that we’re feeding them, the application that drives that particular implementation.”
As publishers continue supplying their AI tools with more data, regulatory and ethical questions around data privacy remain a top concern. Publishers will have to continue juggling competing demands — adhering to ever-changing data privacy laws, respecting consumer wishes for data transparency while serving curated content and navigating how much content personalization is too much — as they expand their use of NLP and data-driven personalization.